Exploring AI's Perception of Educators: A Deep Dive
Written on
Chapter 1: Understanding AI's Vision of Society
In "Our World in AI," we delve into the way Artificial Intelligence interprets various aspects of society. This analysis involves using AI to create images that represent specific societal scenarios, followed by an examination of these outputs. The central question we address is whether AI mirrors reality accurately or exacerbates existing biases.
To perform this analysis, we provide a detailed prompt that describes a commonplace scene. Precision in these descriptions is crucial, as it enables the AI to generate consistent imagery quickly and facilitates the gathering of relevant real-world data. We then analyze the first 40 generated images and juxtapose the results against reality. For today's exploration, our prompt is: “a school teacher in England assisting a student.” We utilized OpenAI’s DALL-E 2 and the open-source Stable Diffusion for this task.
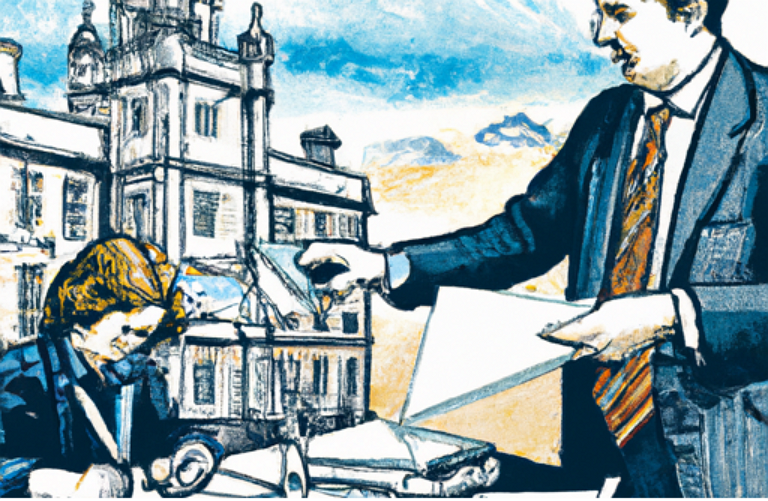
Now, let’s examine the output from Stable Diffusion first. It produced seven monochrome images. Notably, when we adjusted the prompt to depict the teacher sitting at a desk, the resulting images also appeared in a vintage photography style. Among these, our favorite was the fourth image in the first row, showcasing a casual hand-in-pocket stance. However, a significant observation is that all the depicted teachers were female.
DALL-E, on the other hand, displayed more gender diversity, generating images that included seven male teachers. We will now compare these findings with real-world data sourced from the UK Government's school teacher workforce report for the academic year 2021/22, as illustrated in the following graph.
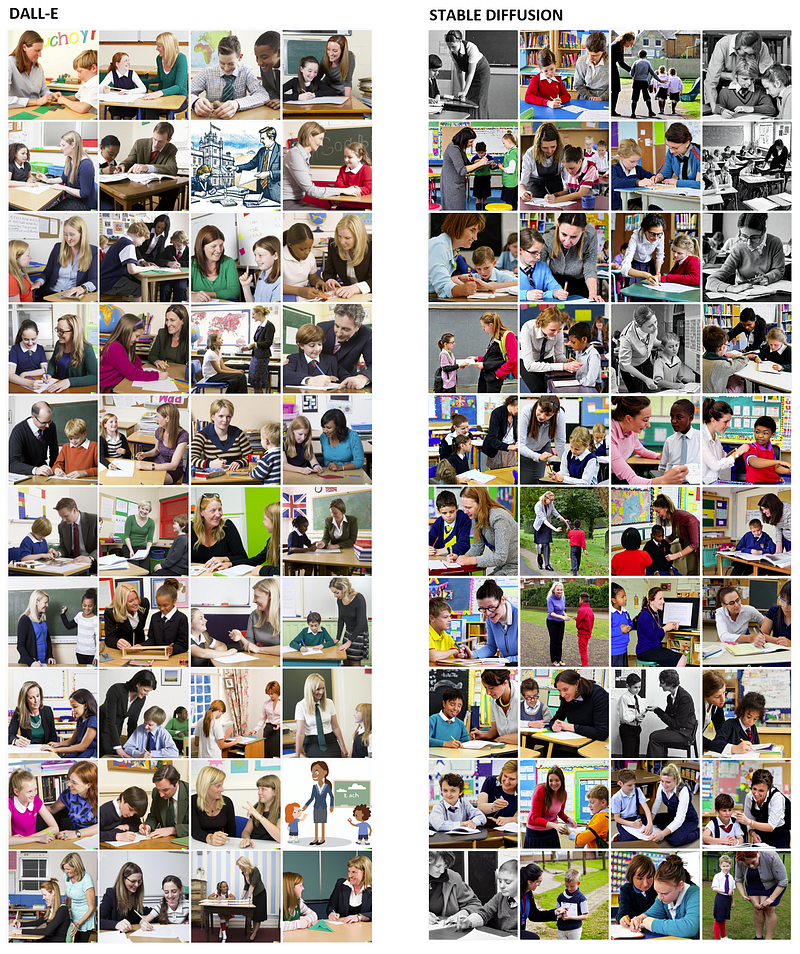
DALL-E's performance was impressive, as the gender distribution among the generated teachers closely matched real-world statistics. Now, we reach the concluding section of this analysis where we evaluate whether AI's representation of society is timely, outdated, or current.
Today's conclusion: DALL-E is categorized as current, while Stable Diffusion is considered outdated in this instance. DALL-E successfully mirrored reality, whereas Stable Diffusion exhibited a tendency to reinforce biases with this particular prompt. Due to heavy traffic on the Stable Diffusion Playground server during data collection, some anomalies appeared in the images. We intend to revisit this analysis at a later date. For now, we are pleased with DALL-E's results!
Next week in "Our World in AI": a look at professors.
In our statistical examination, we conduct a Chi-Square test to assess the independence of gender concerning the data source. The null hypothesis posits no correlation between gender and the data source, while the alternative suggests a relationship exists. Our interpretation is as follows: rejecting the null hypothesis indicates a connection, allowing us to identify the source, while failing to reject implies no discernible relationship. Our significance threshold is set at α = 0.10. DALL-E's comparison with real-world data yielded a p-value of 0.585 (do not reject).
Chapter 2: Insights from Experts
This video, titled "Episode 208: Exploring AI's Impact in the Classroom," delves into how AI technologies are shaping the educational landscape and the implications for teachers and students alike.
In this insightful discussion, "The future of AI in schools with John Hattie, Dylan Wiliam, Arran Hamilton," experts share their thoughts on the evolving role of AI in educational settings and its potential impact on teaching methodologies.